Tables
Turn the numbers from the computer printout into tables
Except for the correlation matrix that is part of the reliability
analysis, you will be required to make new tables, rather than simply
xeroxing the output you get from the computer. This is to indicate
to me that you KNOW what numbers you are looking at to answer the various
questions in the report. It would be useful to bring "blank"
tables for your variables set up like those in the example to class on
the date indicated, so that you can copy your data into them. Tables should
be numbered sequentially so you can refer to them in your report, should
have titles, and should include labels for the variables so that a person
can understand what the numbers in the table are without having to read
the rest of your paper. BE SURE TO DOUBLE- AND TRIPLE-CHECK YOUR COPYING
OF NUMBERS FROM THE PRINTOUT INTO THE TABLES after the class.
Inspect Your Data
- Frequency distributions. There are several useful things to inspect
the frequencies for.
- Problems of low variability. If 80% or more of the respondents
chose one answer for an item, the variability of that variable is
so low that statistical results with it are extremely problematic
given small samples sizes. Results from such variables cannot be trusted,
and they are often simply discarded.
- Lesser problems of low variability, where 60% or more of the cases
choose one response, or where 80% or more of the cases are in two
adjacent responses (of a variable with more than three responses).
These may just indicate a skew in the population, but might also indicate
a biased sample or a biased question.
- Comparisons across the different questions measuring the dependent
variable, to see which items elicit the most favorable responses,
and which the least favorable.
- Information about the distribution of attitudes in the population.
Because your samples are non-random, you have to interpret these results
very cautiously, but it is still interesting to find out what people
said.
- Reliability analysis and correlations among the questions measuring
the dependent variable. Ideally, the reliability analysis should show
all of the corrected item-total correlations (the correlation of each
item with the sum of all the other items) to be moderately high and
positive (better than .4 or .5). and the coefficient alphas to be over
.7. Also, for each item, the output should state that removing
the item would lead to a lower alpha. If all of these things
are true, your items are all good.
If you don't have the ideal, there are several possible patterns to
check for in the correlation matrix itself:
- One or two questions show low negative correlations and small positive
ones with the other items; these questions have a low item-total correlation
and an indication that alpha will go UP if they are removed. This
probably means those questions are "bad" and the others
are OK. You discard the bad questions and get a revised index using
only the good ones.
- There are subsets of questions that have moderate or strong positive
correlations with each other but negative or weak correlations with
the others. This means the questions seem to be tapping more than
one conceptual variable. You try to see which set most captures what
you had in mind and use those for your index. Occasionally, you decide
that both sets are interesting, and create two indices, one for each.
- There are a lot of strong negative correlations for one or two
variables, and their item-total correlations are strongly negative.
This usually means that you have forgotten to "reverse score"
a question, or that subjects read the opposite meaning into a question
than you intended. SEE ME.
- Most correlations in the table are close to zero, and negative
ones are scattered across different questions, alphas and item-total
correlations are low. This means that none of the items are properly
related to any of the others, that there really is no single concept
that your questions measure. This is the worst situation to be in,
but it is rare. Most often, this turns out to be due to mistakes in
coding the data, usually when partners code their data separately
and turn out to be doing it differently. If you have data like this
and you have checked for and ruled out coding errors, you definitely
need to see me.
- Look to see if the means of the INDEX get larger in the expected
direction across categories of the OPEN-ENDED question, and that the
analysis of variance test is significant at p < .01 or smaller. This
should occur if the INDEX and the OPEN-ENDED question are both "valid"
measures of your concept. If this does not happen, you try to figure
out which measure is "bad." Keep in mind that the open-ended
question might have a problem, or that the open-ended categories may
be out of order.
- Tests of the "obvious hypothesis using the index will employ
either correlation analysis (if your independent variable is
continuous) or analyses of variance (if I.V. is categorical). Tests
of the hypothesis using the open-ended question will use chi-squares.
Look to see if the hypothesis is confirmed or not. You may get
- significant findings using both measures,
- nothing using either measure, or
- significant findings with one measure and not with the other.
Again, there are implications for the validity of each of these measures
from these results. We will discuss how you interpret these in class.
The following are a set of tables, based on the student questionnaire
presented a few pages back. In class you will be given the actual printouts
from these data, just like the printouts you will later receive for your
own data. After the tables, there is a guide to the output, titled "Numbers
are our friends."
EXAMPLE TABLES for Univariate statistics. The computer printout
provides a lot of statistics. The first few pages go into Tables 1 and
2.
Table 1. Frequency Distributions for Independent Variables
(I show only four items for brevity of presentation)
4sex31 |
freq |
party4 |
freq |
relig5 |
freq |
libcon9 |
freq |
male |
30 |
Repub |
22 |
Catholic |
18 |
Ext. Liberal |
1 |
female |
29 |
Dem |
16 |
Jewish |
1 |
2 |
10 |
|
|
Prog |
3 |
Protestant |
14 |
3 |
10 |
|
|
Ind |
9 |
Buddhist |
1 |
4 |
1 |
|
|
Green |
1 |
Hindu |
1 |
5 |
6 |
|
|
Libertar |
1 |
Deist |
2 |
6 |
9 |
|
|
Other |
7 |
None |
19 |
7 |
5 |
|
|
|
|
Other |
3 |
8 |
5 |
|
|
|
|
|
|
9 |
7 |
|
|
|
|
|
|
Ext. Conserv. |
1 |
Note: The computer prints only values of the variable that have
been chosen by respondents in the frequency tables. You should put in
all values because it helps you to compare the questions in terms of where
people feel most favorable or unfavorable. Note that the tables do NOT
report a mean for the open-ended question, or the independent variables,
even though the computer printed them, because in most cases it would
be meaningless.
Table 2. Frequency Distributions And Means for Dependent Variables.
(I present only the first four variables and the OPEN code for brevity)
deter11 |
f |
state12 |
f |
safe13 |
f |
cand14 |
f |
OPEN1 |
f |
1 |
11 |
1 |
2 |
1 |
26 |
1 |
5 |
strongly opopose |
22 |
2 |
19 |
2 |
8 |
2 |
6 |
2 |
11 |
mod. oppose |
5 |
3 |
5 |
3 |
11 |
3 |
6 |
3 |
9 |
ambig. |
8 |
4 |
8 |
4 |
7 |
4 |
5 |
4 |
6 |
mod. favor |
10 |
5 |
12 |
5 |
19 |
5 |
9 |
5 |
9 |
strongly favor |
14 |
6 |
4 |
6 |
12 |
6 |
7 |
6 |
19 |
|
|
|
|
|
mean |
3.05 |
|
4.17 |
|
2.76 |
|
4.02 |
|
(inapp.) |
Reliability analysis; correlations among the closed-ended questions.
The computer next printed out a triangular correlation matrix, which
you copy into Table 3. (You may paste the matrix, as I have here, but
you must provide a title.)
Table 3: Correlations among closed-ended questions and with the total
scale
|
deter11 |
safe13 |
cand14 |
retrib15 |
wrong17 |
cheap18 |
deter11 |
1.0000 |
|
|
|
|
|
safe13 |
.613 |
1.0000 |
|
|
|
|
cand14 |
.505 |
.648 |
1.0000 |
|
|
|
retrib15 |
.533 |
.522 |
.609 |
1.0000 |
|
|
wrong17 |
.470 |
.627 |
.560 |
.559 |
1.0000 |
|
cheap18 |
.307 |
.516 |
.550 |
.561 |
.477 |
1.0000 |
fed19 |
.085 |
.249 |
.213 |
-.151 |
-.083 |
-.098 |
moral20 |
.669 |
.743 |
.714 |
.737 |
.692 |
.676 |
final21 |
.660 |
.623 |
.604 |
.710 |
.638 |
.523 |
apply22 |
.548 |
.511 |
.476 |
.380 |
.543 |
.262 |
|
fed19 |
moral20 |
final21 |
apply22 |
fed19 |
1.0000 |
|
|
|
moral20 |
.054 |
1.0000 |
|
|
final21 |
-.023 |
.846 |
1.0000 |
|
apply22 |
.158 |
.494 |
.546 |
1.0000 |
N of Cases= 59.0
Item-total Statistics
|
Scale Mean If Item Deleted |
Scale Variance if Item Deleted |
Corrected Item-Total Correlation |
Squared Multiple Correlation |
Alpha if Item Deleted |
deter11 |
29.1844 |
135.506 |
.680 |
.583 |
.892 |
safe13 |
29.4725 |
126.763 |
.784 |
.669 |
.884 |
cand14 |
28.2183 |
130.151 |
.758 |
.610 |
.886 |
retrib15 |
28.7683 |
128.557 |
.693 |
.632 |
.891 |
wrong17 |
28.6420 |
135.123 |
.698 |
.601 |
.891 |
cheap18 |
29.7268 |
138.933 |
.582 |
.547 |
.898 |
fed19 |
29.2692 |
159.576 |
.057 |
.332 |
.924 |
moral20 |
29.3878 |
124.042 |
.895 |
.865 |
.877 |
final21 |
29.0827 |
127.008 |
.808 |
.767 |
.883 |
apply22 |
29.3653 |
137.301 |
.592 |
.467 |
.897 |
Reliability Analysis - Scale (Alpha)
Reliability Coefficients 10 Items
Cronback's Alpha=.903, Standardized Item Alpha=.898
We will discuss in class how you would interpret these numbers and what
are their implications for validity.
Bivariate association between open-ended question and index. The
computer gives you the mean for the index separately for each category
of the open-ended question.
Table 4. Mean of the Index for Each Category of the Open-ended Question.
OPEN1 |
N |
Mean
|
Standard
Deviation
|
f |
p-value |
Strongly Oppose |
22 |
21.65 |
5.547 |
|
|
Mod. Oppose |
5 |
25.00 |
5.788 |
33.45 |
.001 |
Amabiguous |
8 |
30.94 |
13.157 |
|
|
Mod. Favor |
10 |
37.10 |
6.280 |
|
|
Strongly Favor |
14 |
48.71 |
5.470 |
|
|
Tests of "obvious" hypothesis and other hypotheses.
Table 5 a. Mean of Index Separately by Political Party Preference (Obvious hypothesis).
partycat |
Mean Index |
N |
Standard Dev. |
F |
p-value |
Repub. or libertarian |
41.55 |
23 |
10.93 |
8.872 |
.001 |
Democrat |
27.00 |
16 |
12.23 |
|
|
Prog. or green |
18.58 |
4 |
3.47 |
|
|
Independ |
24.11 |
9 |
6.57 |
|
|
Other |
31.86 |
7 |
9.05 |
|
|
Table 5 b. Mean of Index Separately by Religion
religcat |
Mean Index |
N |
Standard Dev. |
F |
p-value |
Catholic |
35.22 |
18 |
10.47 |
4.937 |
.004 |
Protestant |
40.00 |
14 |
13.83 |
|
|
None |
25.47 |
19 |
10.93 |
|
|
Deist, Jewish, Hindu, Buddhist, Other |
28 |
8 |
11.90 |
|
|
Table 6 a. Test of obvious hypothesis using open-ended question. Percentages of subjects in each category of the open-ended
coding categories (collapsed) in relationship to political party (further collapsed).
|
OPEN (grouped) |
Party (trichotomy) |
Codes 1-2 (Oppose) |
Code 3 (Ambiguous) |
Code 4-5 (Favor) |
Repub. + Libertar (23) |
13% (3) |
43.5% (10) |
43.5% (10) |
Democrat(16) |
56.3% (9) |
25% (4) |
45% (9) |
other (20) |
50% (10) |
45% (9) |
5% (1) |
Chi-square; p-value |
14.047; p< .007 |
Table 6 b. Test of second hypothesis, using open-ended answers. Percentage of respondents in each category of the open-ended question (collapsed) by categories of religion.
|
OPEN (grouped) |
Religion (four categories) |
Codes 1-2 (Oppose) |
Code 3 (Ambiguous) |
Codes 4-5 (Support) |
Catholic |
27.8% (5) |
50% (9) |
22.2% (4) |
Protestant |
28.6% (4) |
14.3% (2) |
57.1% (8) |
None |
42.1% (8) |
52.6% (10) |
5.3% (1) |
Deist, Jewish, Hindu, Buddhist, other |
62.5% (5) |
25% (2) |
12.5% (1) |
Chi-square, p-value |
16.024; p <.014 |
*** END OF EXAMPLE***
NUMBERS ARE OUR FRIENDS: A BRIEF STATISTICS GUIDE
Some statistical concepts:
- Pearson product-moment correlations, analysis of variance, and chi-square
are three of many ways to calculate the likelihood that patterns of
relationships between variables are unlikely to be due to chance.
- Correlations can run from +1.00 through 0 to -1.00. Correlations
indicate the extent to which one variable is associated with a second.
Alternatively, one can see it as the extent to which you can predict
one variable from the other. The larger the number, the better the prediction.
The significance of a correlation -- the likelihood that it represents
a "real" relationship between the two variables -- can be
calculated. It is based in part on the size of the correlation and in
part upon the sample size.
- Analysis of variance (and t-test, a special case involving only two
sample groups) tests whether it is reasonable to assume that the sample
means on a continuous variable for several different groups come from
different populations. That is, can we conclude with some confidence
that there is something about the groups that is "really"
different, as opposed to having to accept that the apparent mean differences
are due to chance.
- Chi-square tests whether the distribution of cases in a contingency
table (a cross-tabulations of two nominal or ordinal variables) deviates
from what we would expect the distribution of cases in the table would
be based on chance alone.
- These tests of "significance" are based on ratios that
are calculated and then looked up in tables. (Nowadays, the computer
does all of this for you.) You need not understand the calculations
to understand the concept. What the level of significance tells you
is what proportion of times you would expect to find as strong a relationship
as is indicated by the data simply by the operation of chance factors.
The larger the obtained ratio, and the smaller the p-value, the less
likely it is that only chance is operating, and, therefore, the more
likely it is that the relationship represents some systematic association
between the variables being tested.
- We do not say that we have "proved" that a relationship
exists, because we can only make probabilistic statements. The size
of the p-value gives us only more or less confidence that the
relationships we observe represent something meaningful.
Next Section
Top
Questions? Comments? Please contact jpiliavi@ssc.wisc.edu
|
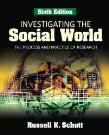
Home
Vita
Sociology 236
Sociology 357
Sociology 647
Sociology 965
Sociology Homepage
|